Datasets
Binaries/Code | Datasets | Open Source Software |
Human Part Segmentation Datasets
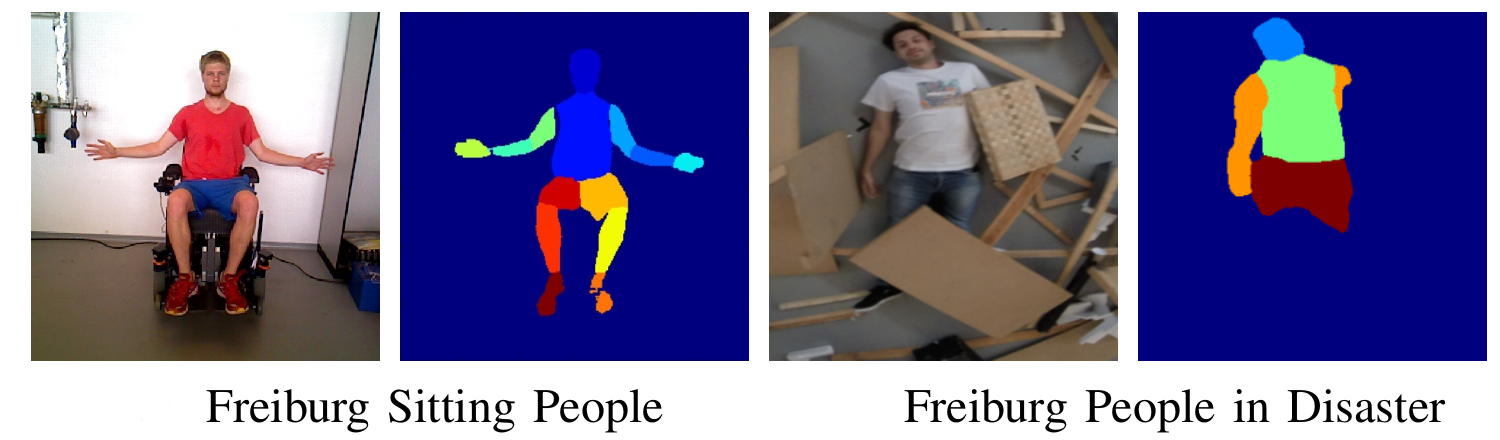
Terms of use
The dataset is provided for research purposes. Any commercial use is prohibited. When using the dataset in your research work, you should cite the following paper:Gabriel L. Oliveira, A. Valada, C. Bollen, W. Burgard, Thomas Brox: Deep Learning for Human Part Discovery in Images, IEEE International Conference on Robotics and Automation (ICRA), 2016.
We introduced two new datasets for human semantic part segmentation: Freiburg Sitting People and Freiburg People in Disaster. They provide high resolution data for experiments on ground and aerial robot segmentation applications.
Freiburg Sitting people constitutes a dataset with two hundred and one images of six different people in multiple viewpoints and in a wide range of orientations. The data is segmented into 14 parts. Download Freiburg Sitting Dataset (2.3MB)
Freiburg People in Disaster dataset is a collection of images captured using an aerial platform. The dataset consists of images and corresponding segmentation masks in an environment that mimics disaster scenario, with clutter and heavy occlusion around. For this specific dataset only a set of images are publicly available with its correspondent segmentation mask. Download Disaster Dataset (17.6MB)
Range Part Segmentation Dataset
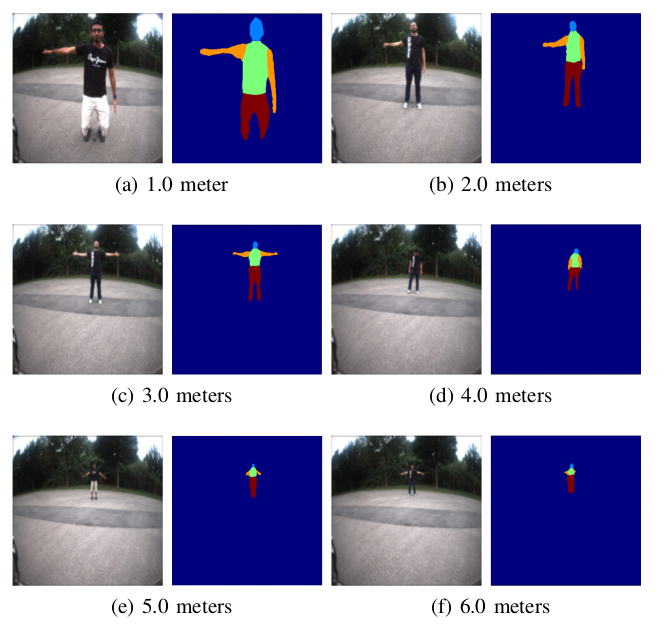
Terms of use
The dataset is provided for research purposes. Any commercial use is prohibited. When using the dataset in your research work, you should cite the following paper:Gabriel L. Oliveira, W. Burgard, Thomas Brox: Efficient Deep Methods for Monocular Road Segmentation, IEEE/RSJ International Conference on Intelligent Robots and Systems (IROS), 2016.
We introduced a new robotics datasets in order to measure range robustness in the human part segmentation task. The dataset has two persons on distances ranging from 0.8m to 6.0m, capturing images every 20cm. Range Part Segmentation Dataset (2.4MB)