Deep learning for automated image analysis in generic imaging modalities
![]() |
Project within the BIOSS Cluster of Excellence (EXC 294) |
Project members
Prof. Dr. Thomas BroxDr. Thorsten Falk
Maxim Tatarchenko
Abstract
We will develop new deep learning methods in order to cover a broader set of tasks in biomedical data analysis. Concrete challenges to be approached will be the full applicability of deep learning to image sequences and volumetric images. We will also work on the challenge to obtain good results with little training data given for a particular task. To this end, we will investigate the relationship between deep networks trained for different tasks and a generic deep network trained on all of them jointly.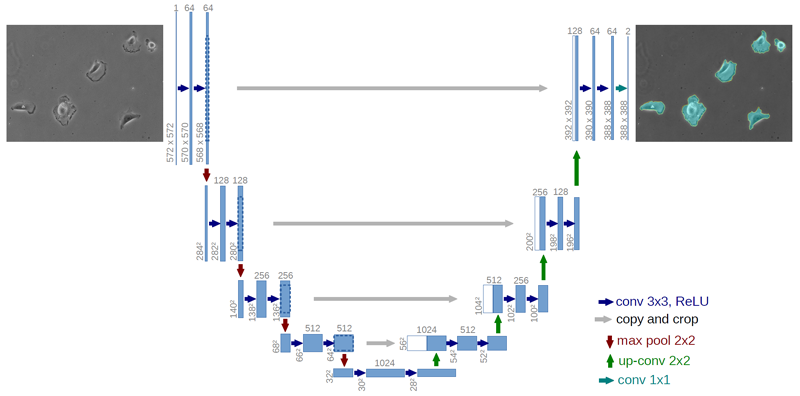
Image segmentation with a deep network. The network can be trained for many similar biomedical segmentation tasks. In this project, we want to extend the framework to image sequences and volumetric images. |
Publications
Medical Image Computing and Computer-Assisted Intervention (MICCAI), Springer, LNCS, Vol.9901: 424--432, Oct 2016
Medical Image Computing and Computer-Assisted Intervention (MICCAI), Springer, LNCS, Vol.9351: 234--241, 2015